ABSTRACT:
Using a lagged polynomial regression model, which used COVID-19 data from 2020 with no vaccines, the prediction of COVID-19 was performed in a scenario with vaccine administration for Tucumán in 2021. The modeling included the identification of a contagion breaking point between both series with the best correlation. Previously, the lag that served to obtain the smallest error between the expected and observed values was indicated by means of cross correlation. The validation of the model was carried out with real data. In 21 days, 18,640 COVID-19 cases out of 20,400 reported cases were predicted. The maximum peak of COVID-19 was estimated 21 days in advance with the expected intensity.
Keywords:
Forecasting; Model; COVID-19; Vaccines
INTRODUCTION
In March 2020, the World Health Organization (WHO) declared the coronavirus disease (COVID-19) a pandemic11. World Health Organization. Coronavirus disease 2019 (COVID-19) situation report, 51. Genebra: World Health Organization; 2020. [accedido el 5 jun. 2021]. Disponible el: https://apps.who.int/iris/bitstream/handle/10665/331475/nCoVsitrep11Mar2020-eng.pdf?sequence=1&isAllowed=y
https://apps.who.int/iris/bitstream/hand... . It urged the activation of various protocols to contain its spread22. World Health Organization. Report of the WHO-China Joint Mission on Coronavirus Disease 2019 (COVID-19). Genebra: World Health Organization; 2020. [accedido el 5 jun. 2021]. Disponible el: https://www.who.int/docs/default-source/coronaviruse/who-china-joint-mission-on-covid-19-final-report.pdf
https://www.who.int/docs/default-source/... . In Argentina, the first case was detected in March 2020 in Buenos Aires, declaring mandatory quarantine by Decree of Necessity and Urgency33. Argentina. Ministerio de Salud. Decreto de Necesidad y Urgencia 260/2020 [Internet]. 2020. [accedido el 5 jun. 2021]. Disponible el: https://www.argentina.gob.ar/coronavirus/dnu
https://www.argentina.gob.ar/coronavirus... .
At the beginning of 2021, no vaccines had been administered to the population and after the reopening of activities, the second wave of COVID-19 began.
The objective was to predict the trend of COVID-19 cases during 2021 for a scenario with vaccine administration and its maximum peak, studying the statistical behavior of COVID-19 data in 2020 without the application of vaccines.
METHODS
The study was carried out in the province of Tucumán, in northwestern Argentina, which was chosen due to the lack of prediction of COVID-19 cases and for being the second most densely populated province in the country, with reported 1,338, 523 inhabitants44. Instituto Nacional de Estadística y Censo. República Argentina. Censo Nacional de Población, Hogares y Viviendas 2010. [Internet]. 2020 [accedido el 21 may. 2021]. Disponible el: https://www.indec.gob.ar/indec/web/Nivel4-CensoProvincia-3-999-90-000-2010
https://www.indec.gob.ar/indec/web/Nivel... .
The elaboration of the prediction model for cases of COVID-19 consisted of identifying in data of COVID-19 of 2020 a lag of days t that best correlates with a lag of days t of COVID-19 of 2021, using as reference a point break of infections in the first series. This being identified, a cross-correlation was performed between the lags, in order to find the best one to fit the data with a lagged polynomial regression model and predict the current COVID-19 trend.
Data conversion: an order-one differencing was used to stabilize the mean and reduce the trend. The p value was calculated with the t statistic with n-2 degrees of freedom and with n based on the number of samples that overlap in the cross-correlations. The analysis was performed with Past 3.2255. Hammer Ø, Harper DAT, Ryan PD. 2001. PAST: Paleontological software package for education and data analysis. Paleontological Electronica 4(1):9. Disponible el: https://www.nhm.uio.no/english/research/infrastructure/past/
https://www.nhm.uio.no/english/research/... ,66. Covid19-prediction. COVID-19 prediction of tendency for 2021 in northwestern Argentina. Disponible el: https://github.com/Agustino216/Covid19-prediction
https://github.com/Agustino216/Covid19-p... .
Two COVID-19 data sets were used, which were published daily by the Ministry of Public Health of the province of Tucumán (Ministerio de Salud Pública de la provincia de Tucumán – MSPT)77. Gobierno de Tucumán. Ministerio de Salud Pública. [Internet]. 2020. [accedido el 29 may 2021]. Disponible el: https://msptucuman.gov.ar/category/noticias/
https://msptucuman.gov.ar/category/notic... . The first set from 03/18/2020 to 11/27/2020. The second set from 03/19/2021 to 05/20/2021. A matrix of lags for COVID-19 of 2020 with different amounts of days in length was created. Previously, the start and end dates of the lags were obtained based on a contagion breakpoint, indicated by a 50% increase in all reported cases before the peak of COVID-19 in 2020. A 15-day moving average was used. The length in t days of the lags (l) were explored at 30, 35, 40, and 45 days.
The identification of the lag of COVID-19 2020 to elaborate the training set was determined by Pearson’s correlation (rp) with p>0.05 with the lag of COVID-19 of 2021. Once the lag was identified, a cross-correlation was performed (rd) with p>0.05 between them. Thus, the location in the predictive series yi (COVID-19 2020) was obtained for its best delay m. In Appendix 1, it is indicated by means of a flow diagram to the methodology used in details.
Model used: the 2020 COVID-19 lag identified in the m delay together with the 2021 COVID-19 data lag were fitted with a lagged polynomial regression model. This type of model was used because COVID-19 cases are random and non-linear. The Polynomial88. Cromwell JB, Labys WA, Hannan MJ, Terraza M. Multivariate tests for time series models. USA: SAGE University paper. regression model used was:
Where xi represents the differentiated and predicted COVID-19 cases for 2021 on day i, a, b, c are coefficients of the polynomial model, y is the 2020 COVID-19 predictive series on day i that best predicts x on function of y for its best lag i-m, while e represents the estimated error. The process was invertible for the differentiation performed. The autocorrelation of the residuals of the best model was null. The evaluation of the model was carried out with real data from COVID-19, using the mean absolute percentage error (MAPE). A forecast horizon was subsequently assessed in the same way.
RESULTS
The results indicated that the break point for COVID-19 infections in 2020 was 09/25/2020 (692 cases), while in 2021 it was 05/13/2021 (723 cases).
The COVID-19 data lag in 2021 used to build the model was from 03/30/2021 to 05/13/2021 and for the COVID-19 data lag in 2020 it was from 08/18/2020 to 01/ 10/2020. Between the series, rp= -0.296 was obtained, with p=0.04, while for a delay of m=17 days it was rd=0.488 with p=0.008 (Figure 1). The model obtained for this delay was: xi=-1.935E-06 y33. Argentina. Ministerio de Salud. Decreto de Necesidad y Urgencia 260/2020 [Internet]. 2020. [accedido el 5 jun. 2021]. Disponible el: https://www.argentina.gob.ar/coronavirus/dnu
https://www.argentina.gob.ar/coronavirus... i-m+0.0006216 y22. World Health Organization. Report of the WHO-China Joint Mission on Coronavirus Disease 2019 (COVID-19). Genebra: World Health Organization; 2020. [accedido el 5 jun. 2021]. Disponible el: https://www.who.int/docs/default-source/coronaviruse/who-china-joint-mission-on-covid-19-final-report.pdf
https://www.who.int/docs/default-source/... i-m+0.02296 yi-m+11.44, with R22. World Health Organization. Report of the WHO-China Joint Mission on Coronavirus Disease 2019 (COVID-19). Genebra: World Health Organization; 2020. [accedido el 5 jun. 2021]. Disponible el: https://www.who.int/docs/default-source/coronaviruse/who-china-joint-mission-on-covid-19-final-report.pdf
https://www.who.int/docs/default-source/... =0.315, F=36.8, p= 0.026 (Figure 2). The autocorrelation of the residuals was null.
The 17 predicted days were from 05/14/2021 to 05/30/2021 (n=17) (Figure 2) with 14,042 predicted cases out of 15,824 reported ones, MAPE was 11.3. The maximum variability of COVID-19 cases from 05/31/2021 to 06/03/21, 4,576 predicted cases accumulated over 4,598 reported cases with MAPE of 0.47. The maximum peak of COVID-19 at 21 days was estimated for 06/03/2021 with 1,200 cases and occurred on 06/04/202 with 1,485 cases.
DISCUSSION
The results showed that the model underestimated the number of events occurred before 05/27/2021, the moment in which strict social restrictions were installed99. Gobierno de Tucumán. Comité Operativo de Emergencia de Tucumán. 2021. [Internet] [accedido el 22 may 2021]. Disponible el: https://coe.tucuman.gov.ar/recursos/documentos/archivos/archivo_333_20210522110758.pdf
https://coe.tucuman.gov.ar/recursos/docu... and the real cases were accompanied toward the maximum peak when the restrictions were installed. It is possible that underestimation is influenced by society’s relaxation regarding the administration of vaccines. Before starting the model on 04/22/2021, the vaccination campaign accumulated 230,000 applied doses1010. Gobierno de Tucumán. Ministerio de Salud Pública. Llegaron 24600 dosis de Sputnik V a la provincia [Internet]. 2021. [accedido el 31 oct. 2021]. Disponible el: https://vacunartuc.gob.ar/llegaron-24600-dosis-de-sputnik-v-a-la-provincia/
https://vacunartuc.gob.ar/llegaron-24600... . While two days after the first peak of COVID-19, on 05/06/2021, the application of 306,000 doses was accumulated1111. Gobierno de Tucumán. Ministerio de Salud Pública. Desde el inicio de la campaña Tucumán lleva aplicadas 306.833 dosis de vacunas contra el Covid-19. [Internet]. 2021. [accedido el 31 oct. 2021]. Disponible el: https://vacunartuc.gob.ar/desde-el-inicio-de-la-campana-tucuman-lleva-aplicadas-306-833-dosis-de-vacunas-contra-el-covid-19/
https://vacunartuc.gob.ar/desde-el-inici... . Another form of underestimation of the model would be the absence of social restrictions. We jointly compared the increase in COVID-19 cases reported by the MSPT, those predicted, and an Index of Movement of People in Supermarkets and Pharmacies1212. Google COVID-19 Community Mobility Reports. [Internet]. 2021. [accedido el 01 nov. 2021]. Disponible el: https://www.google.com/covid19/mobility/
https://www.google.com/covid19/mobility/... and we observed that they behaved in a similar way (Figure 2).
The accuracy of the model is similar to that of other reported investigations, such as the one calculated with a parsimonious and robust survival and convolution model1313. Wang Q, Xie S, Wang Y, Zeng D. Survival-convolution models for predicting COVID-19 cases and assessing effects of mitigation strategies. Front Public Health 2020; 8: 325. https://doi.org/10.1101%2F2020.04.16.20067306
https://doi.org/https://doi.org/10.1101%... . The duration of the prediction obtained is similar to that achieved with the extended susceptible-exposed-infectious-recovered model1414. Ghostine R, Gharamti M, Hassrouny S, Hoteit I. An extended SEIR model with vaccination for forecasting the COVID-19 pandemic in Saudi Arabia using an ensemble kalman filter. Mathematics 2021; 9(6): 636. https://doi.org/10.3390/math9060636
https://doi.org/https://doi.org/10.3390/... .
The model presented here was able to predict the trend in the dynamics of expected COVID-19 cases toward the maximum peak. However, it was only able to predict the COVID-19 peak for June 3rd, with two COVID-19 peaks actually occurring in 2021, one on 06/04/21 and the other on 06/08/21.
In conclusion, we highlight that the trends of COVID-19 cases in 2021 in Tucumán could be predicted by analyzing the statistical behavior of the first wave of COVID-19 that occurred in 2020.
Appendix 1. Flow diagram of the proposed model for the prediction of COVID-19 in Tucumán.
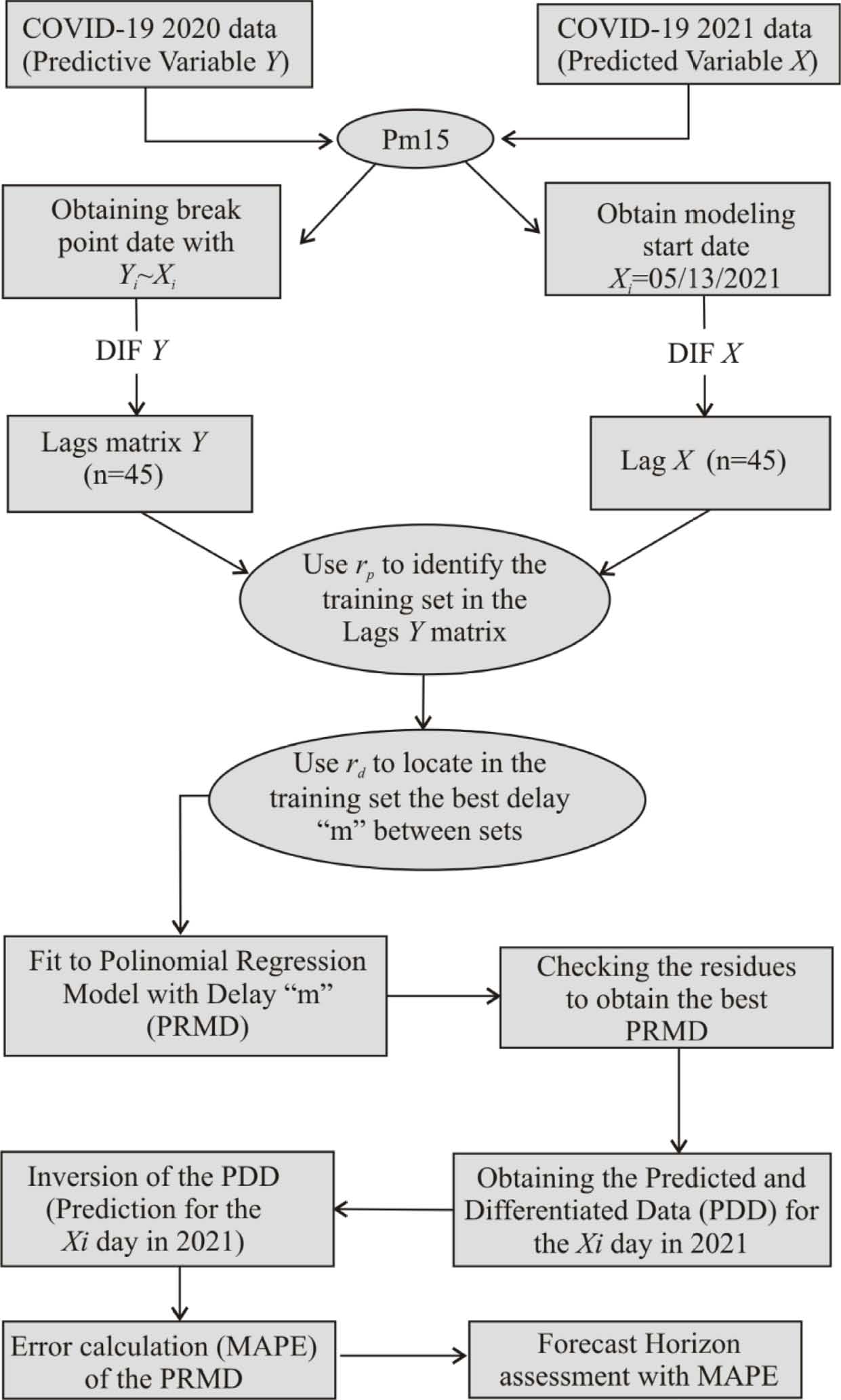
MAPE: mean absolute percentage error, Mean: 15-day mobile mean, DIF: Differentiation of single order data.
References
- 1.World Health Organization. Coronavirus disease 2019 (COVID-19) situation report, 51. Genebra: World Health Organization; 2020. [accedido el 5 jun. 2021]. Disponible el: https://apps.who.int/iris/bitstream/handle/10665/331475/nCoVsitrep11Mar2020-eng.pdf?sequence=1&isAllowed=y
» https://apps.who.int/iris/bitstream/handle/10665/331475/nCoVsitrep11Mar2020-eng.pdf?sequence=1&isAllowed=y - 2.World Health Organization. Report of the WHO-China Joint Mission on Coronavirus Disease 2019 (COVID-19). Genebra: World Health Organization; 2020. [accedido el 5 jun. 2021]. Disponible el: https://www.who.int/docs/default-source/coronaviruse/who-china-joint-mission-on-covid-19-final-report.pdf
» https://www.who.int/docs/default-source/coronaviruse/who-china-joint-mission-on-covid-19-final-report.pdf - 3.Argentina. Ministerio de Salud. Decreto de Necesidad y Urgencia 260/2020 [Internet]. 2020. [accedido el 5 jun. 2021]. Disponible el: https://www.argentina.gob.ar/coronavirus/dnu
» https://www.argentina.gob.ar/coronavirus/dnu - 4.Instituto Nacional de Estadística y Censo. República Argentina. Censo Nacional de Población, Hogares y Viviendas 2010. [Internet]. 2020 [accedido el 21 may. 2021]. Disponible el: https://www.indec.gob.ar/indec/web/Nivel4-CensoProvincia-3-999-90-000-2010
» https://www.indec.gob.ar/indec/web/Nivel4-CensoProvincia-3-999-90-000-2010 - 5.Hammer Ø, Harper DAT, Ryan PD. 2001. PAST: Paleontological software package for education and data analysis. Paleontological Electronica 4(1):9. Disponible el: https://www.nhm.uio.no/english/research/infrastructure/past/
» https://www.nhm.uio.no/english/research/infrastructure/past/ - 6.Covid19-prediction. COVID-19 prediction of tendency for 2021 in northwestern Argentina. Disponible el: https://github.com/Agustino216/Covid19-prediction
» https://github.com/Agustino216/Covid19-prediction - 7.Gobierno de Tucumán. Ministerio de Salud Pública. [Internet]. 2020. [accedido el 29 may 2021]. Disponible el: https://msptucuman.gov.ar/category/noticias/
» https://msptucuman.gov.ar/category/noticias/ - 8.Cromwell JB, Labys WA, Hannan MJ, Terraza M. Multivariate tests for time series models. USA: SAGE University paper.
- 9.Gobierno de Tucumán. Comité Operativo de Emergencia de Tucumán. 2021. [Internet] [accedido el 22 may 2021]. Disponible el: https://coe.tucuman.gov.ar/recursos/documentos/archivos/archivo_333_20210522110758.pdf
» https://coe.tucuman.gov.ar/recursos/documentos/archivos/archivo_333_20210522110758.pdf - 10.Gobierno de Tucumán. Ministerio de Salud Pública. Llegaron 24600 dosis de Sputnik V a la provincia [Internet]. 2021. [accedido el 31 oct. 2021]. Disponible el: https://vacunartuc.gob.ar/llegaron-24600-dosis-de-sputnik-v-a-la-provincia/
» https://vacunartuc.gob.ar/llegaron-24600-dosis-de-sputnik-v-a-la-provincia/ - 11.Gobierno de Tucumán. Ministerio de Salud Pública. Desde el inicio de la campaña Tucumán lleva aplicadas 306.833 dosis de vacunas contra el Covid-19. [Internet]. 2021. [accedido el 31 oct. 2021]. Disponible el: https://vacunartuc.gob.ar/desde-el-inicio-de-la-campana-tucuman-lleva-aplicadas-306-833-dosis-de-vacunas-contra-el-covid-19/
» https://vacunartuc.gob.ar/desde-el-inicio-de-la-campana-tucuman-lleva-aplicadas-306-833-dosis-de-vacunas-contra-el-covid-19/ - 12.Google COVID-19 Community Mobility Reports. [Internet]. 2021. [accedido el 01 nov. 2021]. Disponible el: https://www.google.com/covid19/mobility/
» https://www.google.com/covid19/mobility/ - 13.Wang Q, Xie S, Wang Y, Zeng D. Survival-convolution models for predicting COVID-19 cases and assessing effects of mitigation strategies. Front Public Health 2020; 8: 325. https://doi.org/10.1101%2F2020.04.16.20067306
» https://doi.org/https://doi.org/10.1101%2F2020.04.16.20067306 - 14.Ghostine R, Gharamti M, Hassrouny S, Hoteit I. An extended SEIR model with vaccination for forecasting the COVID-19 pandemic in Saudi Arabia using an ensemble kalman filter. Mathematics 2021; 9(6): 636. https://doi.org/10.3390/math9060636
» https://doi.org/https://doi.org/10.3390/math9060636
- Financial support: none.
Publication Dates
- Publication in this collection
14 Mar 2022 - Date of issue
2022
History
- Received
02 Sept 2021 - Reviewed
05 Nov 2021 - Accepted
07 Dec 2021 - Preprint
13 Dec 2021